Learner analytics: Hindsight evaluation at course-level
Abstract
The concept of student engagement is a contentious construct. The task of learner analytics (LA) to meaningfully measure student engagement is therefore complicated both by a lack of agreement over what is being measured and a discomfort or lack of confidence around what collated data might believably indicate. This challenge is made harder by availability, accuracy and reliability of data feeds. The aim of LA would be to collate and share early measures of engagement that can be used predictively to support learners’ experience and outcomes. However, most learner analytics from Higher Education Institutions are descriptive and therefore of limited utility. Where the LA available are descriptive, this paper explores how credible such LA might be when used at course level. This small-scale case study supports an analysis of comprehensive data gathered within and beyond LA for a level 4 cohort in one programme across the 2019-20 academic year. It also draws on data relating to study completion of that cohort, with the benefit of hindsight giving further insights to the utility of LA data available earlier in students’ journeys. Given the actual outcomes for these 2019 starters, that same cohort’s understanding of what constitutes ‘engagement’ is then applied to support insights to their own measurable indicators of interaction and actions that might best enable constructive engagement. Meaningful correlations were noted between use of E-resources and student outcomes and the most significant indicators of risk were found to be extensions, fails and non-submissions for assignments in the first semester of level 4 and average grades <39% by the end of level 4. Study recommendations include supporting better and more confident access to e-literature content and targeting timely interventions at students flagged by the most significant early indicators of risk.
Keywords
Learner analytics, student engagement, attainment, retention, higher education
Resumen
El concepto compromiso del estudiante es controvertido. El empleo de perfiles analíticos del alumnado (PAA) para medir el compromiso del estudiante en su aprendizaje se complica tanto por la falta de acuerdo sobre qué es lo que se está midiendo realmente como por la incomodidad o falta de confianza en torno a lo que los datos cotejados indican de manera creíble. Este reto se convierte en algo más complejo por la escasa disponibilidad y la cuestionable precisión y fiabilidad de los datos. El objetivo de los perfiles analíticos del alumnado es recopilar y compartir datos de participación inicial, que puedan utilizarse de forma predictiva para mejorar la experiencia y resultados posteriores. Sin embargo, la mayoría de los PAA recogidos por las instituciones de educación superior son descriptivos y, por tanto, de limitada utilidad. Este artículo explora la credibilidad de dichos PAA cuando se utilizan a nivel de curso y son exclusivamente descriptivos. Este estudio de caso a pequeña escala ofrece un análisis de datos exhaustivos recopilados dentro y fuera de los PAA para una cohorte de nivel 4 a lo largo del curso académico 2019-20. El trabajo también emplea datos sobre la finalización de los estudios de esa cohorte, lo que permite realizar un análisis retrospectivo que proporciona más información sobre la utilidad de esos PAA en una etapa anterior del itinerario de este alumnado. Teniendo en cuenta los resultados reales de estos estudiantes que comenzaron en 2019, aplicamos su comprensión sobre qué significa compromiso, para explicar sus propios indicadores de interacción y acciones que podrían facilitar un compromiso constructivo. Se observaron correlaciones significativas entre el uso de los recursos electrónicos y los resultados de los estudiantes, y se descubrió que los indicadores de riesgo más significativos eran las prórrogas, los suspensos y la no entrega de trabajos en el primer semestre del nivel 4, así como unas notas medias inferiores al 39% al final del nivel 4. Entre las recomendaciones del estudio se incluye el fomentar un acceso mejor y más seguro a los contenidos de la bibliografía electrónica y ofrecer feedback al alumnado que muestra desde el primer momento indicadores de riesgo.
Palabras clave
analíticas de aprendizaje, compromiso del estudiante, rendimiento, retención, educación superior
Resum
El concepte compromís de l'estudiant és controvertit. L'ús de perfils analítics de l'alumnat (PAA) per a mesurar el compromís de l'estudiant en el seu aprenentatge es complica tant per la falta d'acord sobre què és el que s'està mesurant realment com per la incomoditat o falta de confiança entorn del que les dades acarades indiquen de manera creïble. Aquest repte es converteix en una cosa més complexa per l'escassa disponibilitat i la qüestionable precisió i fiabilitat de les dades. L'objectiu dels perfils analítics de l'alumnat és recopilar i compartir dades de participació inicial, que puguen utilitzar-se de manera predictiva per a millorar l'experiència i resultats posteriors. No obstant això, la majoria dels PAA recollits per les institucions d'educació superior són descriptius i, per tant, de limitada utilitat. Aquest article explora la credibilitat de dites PAA quan s'utilitzen al llarg del curs i són exclusivament descriptives. Aquest estudi de cas a petita escala ofereix una anàlisi de dades exhaustives recopilades dins i fora dels PAA per a una promoció de nivell 4 al llarg del curs acadèmic 2019-20. El treball també empra dades sobre la finalització dels estudis d'aquella promoció, la qual cosa permet realitzar una anàlisi retrospectiva que proporciona més informació sobre la utilitat d'aquelles PAA en una etapa anterior de l'itinerari d'aquest alumnat. Tenint en compte els resultats reals d'aquests estudiants que van començar en 2019, apliquem la seua comprensió sobre què significa "compromís", per a explicar els seus propis indicadors d'interacció i accions que podrien facilitar un compromís constructiu. Es van observar correlacions significatives entre l'ús dels recursos electrònics i els resultats dels estudiants, i es va descobrir que els indicadors de risc més significatius eren les pròrrogues, els suspensos i el no lliurament de treballs en el primer semestre del nivell 4, així com unes notes mitjanes inferiors al 39% al final del nivell 4. Entre les recomanacions de l'estudi s'inclou el fet de fomentar un accés millor i més segur als continguts de la bibliografia electrònica i oferir retroacció (feedback) a l'alumnat que mostra des del primer moment indicadors de risc.
Paraules clau
analítiques d'aprenentatge, compromís de l'estudiant, rendiment, retenció, educació superior
Practitioner notes
What is already known about the topic
-
The literature reflects that Student 'Engagement' is a contentious construct and that what is measurable is more about touch points of interaction and their frequency than any reflection on the quality of engagement.
-
Learner analytics systems (LA) are dependent on the accuracy, reliability and compatibility of data feeds beyond the LA.
-
Learner analytics are used both descriptively and predictively, although the latter less so and with more contention.
-
The increased regulatory focus on student progression and continuation reinforces the need for informed use of student data to support timely and effective interventions.
What this paper adds
-
This paper uses the benefit of hindsight and student voice to identify data most relevant to indicating early risk (of non-completion) across a cohort.
-
The paper draws attention to the particular outcome significance of extensions, fails and non-submissions during Semester one Level 4.
Implications of this research and / or paper
-
Students should be explicitly supported in their use of E-Resources (academic literature) from the start of level 4
-
Any late or non-submission, even a brief approved extension, should be seen as symptomatic of a concern that needs explicit and rapid supportive response.
Introduction
Learner analytics systems (LA) collate data captured through digital interactions from different systems into one, where they may be presented through a visual learner analytics dashboard (LAD) and available to students and relevant Higher Education Institution (HEI) personnel. LA are used in HEI primarily to improve Student Experience (Kika, Duan, & Cao, 2016). They have the potential to positively transform learning by better informing HE colleagues’ support for learning processes (Viberg, Hatakka, Bälter, & Mavroudi, 2018) and empowering students to understand their own data so that they might positively adjust their approach to learning (Susnjak, Suganya-Ramaswami, & Mathrani, 2022). However, whilst descriptive analytics might be interesting in collating a picture of what can be observed, their utility is very limited (Susnjak et al., 2022) yet the push towards predictive analytics risks over-generalisation and the failure to acknowledge individual complexities (Parkes, Benkwitz, Bardy, Myler, & Peters, 2020). However, despite the contention, Student Engagement is a vital concept not just in terms of student experience but also as it relates to retention and progression (Korhonen, Mattsson, Inkinen, & Toom, 2019). The 2022 introduction of new expectations for student outcomes from the Office for Students (OfS) makes accurate predictive analytics even more desirable, since UK HEI are now measured against thresholds that include continuation and completion (Ofs, 2022) and there is an expectation that LA should support those outcomes in HEI (GSU, 2019).
There is a lack of consensus about which data might really relate to student engagement as well as the extent to which that data can be captured by LA (Bassett-Dubsky, 2020). Even the concept of student engagement is a contentious construct (Venn, Park, Palle-Anderson, & Hejmadi, 2020), often driven by availability of data (Bond, Buntins, Bedenlier, Zawacki-Richter, & Kerres, 2020; Dyment, Stone, & Milthorpe, 2020) rather than framing student experiences in ways that make sense to a diversity of individual students (Broughan & Prinsloo, 2020). If the data collated acts to create a singular construct of student engagement (Bassett-Dubsky, 2021), this disempowers students and further marginalises those who already believe they do not align with perceived norms of who belongs and how to belong in HE (Broughan & Prinsloo, 2020).
Recognition of the need for a deeper understanding of a diversity of student experiences may be part of the reason why the potential of LA remains under-realised with current use failing to evidence positive impact on student learning and outcomes or learning support and teaching (Viberg et al., 2018). Or it may be that there is a lack of transparency and understanding about how the data shown in LAD are generated (Susnjak et al., 2022), which would make it hard for users to trust the system and the conclusions they might draw from it. This will be exacerbated if there are problems with data accuracy and reliability of data feeds into the LA system (Bassett-Dubsky, 2020).
At The University of Northampton (UON), as with most other UK HEI, the data pulled into the LA system and LAD are a relatively small proportion of the data within the university systems overall. After a problematic launch in 2019-20, UON’s Learner analytics system was relaunched in the 2022-23 academic year and re-branded (partly to disassociate from challenging earlier experiences) from ‘LEARN’ to ‘MyEngagement’. There was no use of an LA system in the intervening years. For the first four weeks of the 2022-23 academic year, the attendance app was not working, so all attendance data had to be recorded manually. Changes to library systems led to inability to draw data from the library systems into ‘MyEngagement’. Initially, as a major data feed, this meant no overall engagement % was indicated. The calculation of overall engagement was amended so that overall engagement % could still be seen but without this library data feed. By the start of the second semester, physical library loans were feeding through to the LAD but e-books and e-journal access data remained unavailable. Data feed of interactions with the Virtual Learning Environment (VLE) have been captured throughout. The attendance system is now working, and ‘MyEngagement’ is therefore collating attendance and VLE interaction frequency. Although further data are available within the VLE itself about the nature of the VLE use, these are not set up to feed through into the LAD. Data are available about any bookable time with a breadth of learner support colleagues but the systems through which students book time with academic librarians and learning support colleagues are not yet set up to feed through to ‘MyEngagement’ and there are privacy and consent issues to consider around pulling in data about student-booked support for matters such as mental health, additional needs, academic integrity referrals and academic advice (Beetham et al., 2022; Mathrani, Susnjak, Ramaswami, & Barczak, 2021).
Summers, Higson, and Moores (2020) drew on data from a large cohort of level 4 students in the 2018-19 academic year, during their HEI’s trial year using LA. They suggest that early measures of engagement are predictive both of future engagement behaviour and future academic outcomes. The data feeds driving their analysis were attendance (both in the form of ‘live’ presence and lecture capture access) and VLE interaction which they analysed retrospectively against end of year grades. They found that students who gain the highest grades have better attendance and use the VLE more. Most significantly, they claim that “it is evident as early as the first 3–4 weeks of the academic year that those students who obtain the highest end-of-year marks are more likely to attend lectures and interact more with the VLE” (Summers et al., 2020)
During the 2019-20 academic year, the University of Northampton similarly trialled LA. Throughout that year, the engagements, achievement and progression of a level 4 cohort (n-30) on one specific programme within the Faculty of Health, Education and Society were measured and explored in collaboration with that student cohort (Bassett-Dubsky, 2020). The data gathered and generated were sourced from a combination of systems, including LA, and (arguably most importantly) directly from the students. The impact of Covid 19 on the 2019-20 data is present but minimal, since it derives predominantly from prior to the National lockdown in March 2020. However, all student data and experience from March 2020 onwards should of course be seen in the context of the pandemic, with the further inequalities in impact that context still brings (Blundell et al., 2022). The majority of this cohort have now left the course, giving an opportunity to further reflect on those initial data with the benefit of hindsight. The aim of this study was to identify any early indicators in the data captured by LA that correlated with student outcomes which might have informed interventions. It explores whether the large-scale patterns and indications seen bySummers et al. (2020) are also evident in the same type of data at programme-level; this relates to generalisability across very different types of programmes as well as the need to retain visibility of individual and diverse student engagements (Gravett, Kinchin, & Winstone, 2020; Zepke, 2018).
However, this paper also aims to consider understandings of what constitutes student ‘engagement’ to evaluate how useful and meaningful this renders LA data. This relevance is then assessed in conjunction with the credibility and reliability of such data feeds as we do and can access; since utility, credibility and reliability directly relate to effectiveness.
Materials and Methods
The University of Northampton is a medium-sized Post-92 UK university, committed to widening participation and with a diverse student body (HESA, 2023).
A mixed-methods approach was taken to gathering and generating in-depth data in a small-scale case study focusing on the entire 2019-20 incoming cohort (n-30) of a single programme within the Faculty of Health, Education and Society. Measurable data were mapped to look for any patterns and contextualised through qualitative focus groups and personal tutor meetings. Ethical approval and consent for the study was sought and gained from the university and participants.
The quantifiable data drawn upon in this paper include attendance, VLE interaction frequency, E-Resource access frequency and grades. Because the card swipe attendance system was not working properly, attendance records were manually captured during the first 11 weeks of the academic year (including Welcome and Induction week) and fed into the attendance system so that attendance could feed through accurately to the LAD.
Further quantifiable and qualitative data were generated throughout the study, beyond that captured by LA (Table 1):
Week commencing |
||||
---|---|---|---|---|
23/09/2019 |
Welcome & Induction week |
Focus group discussions of hopes, worries and pressures, as well as any peer or self-suggestions as to how these pressures might be managed. |
1-2-1 Personal tutorial notes |
Extensions, fails and non- submissions recorded from VLE and Module boards. |
30/09/2019 |
Semester 1 - Week 1 |
Survey exploring expectations and existing support networks. |
||
|
||||
21/10/2019 |
Semester 1 - Week 4 |
Survey rating and explaining current levels of enjoyment and engagement; students’ prior studies and how they felt their prior studies had prepared them for university study. |
||
|
||||
09/12/2019 |
Semester 1 - Week 11 |
Focus groups exploring why students thought there might be a ‘wobble week’ (Morgan, 2019) between weeks 3-6, and again over the Winter break, as well as what students thought were the toughest challenges and how to get through these to stay at university. Diamond-9 ranking activity and focus groups exploring factors the students felt indicated engagement. |
||
|
||||
17/02/2020 |
Semester 2 - Week 4 |
Survey (anonymous). This was comprehensive and measured satisfaction with a range of course and university characteristics, rating their own engagement, financial comfort, mental wellbeing, sense of belonging, readiness for university, how realistic their starting expectations for university seemed now and whether they were first generation university students, mature students, and lived on or off campus. (Gender was not taken into account as the cohort size was small and included only one male.) |
1-2-1 Personal tutorial notes |
Extensions, fails and non- submissions recorded from VLE and Module boards. |
Descriptive and relational analysis of quantitative data was carried out using Excel (though the scale of study was never intended to lend itself to inferential statistics or generalisable claims). These were evaluated alongside the qualitative data, which were analysed using reflective thematic analysis (Braun & Clarke, 2022), with themes identified and refined after a thorough process of familiarisation and coding; explored with peers and students through the study blog (Bassett-Dubsky, 2020) and seminars throughout 2019-20.
Researcher and cohort perception during the 2019-20 year was that the non-LA data were far more relevant in terms of understanding student engagements (as well as building positive relationships through the extensive process of generating those data). However, it was the need to evaluate the meaning and relevance of the LA data that fuelled the extensiveness of those informative and relationship-building conversations; and had some influence on the varying directions of those conversations as the year progressed. Perhaps more with hindsight, the synthesis of those multiple data sources is where useful insights were found, which would suggest a role for LAD if the most relevant data were reliably available and combined, appropriately interpreted, and led to meaningful, timely action (GSU, 2019).
Analysis and Results
End of year grades as confirmed at end of year award boards were grouped by degree classification, so that average grades of 70%+ were classed as First, grades between 60-69% were classed as Two-1, grades between 50-59% were classed as Two-2, grades between 40-49% were classed as Third and fail grades of 39% or lower were unclassified (None).
End of year grades were considered alongside total interactions (as captured by LA between 1/10/2019 and 30/04/2020; including E-resource use, VLE interactions and attendance) Figure 1).
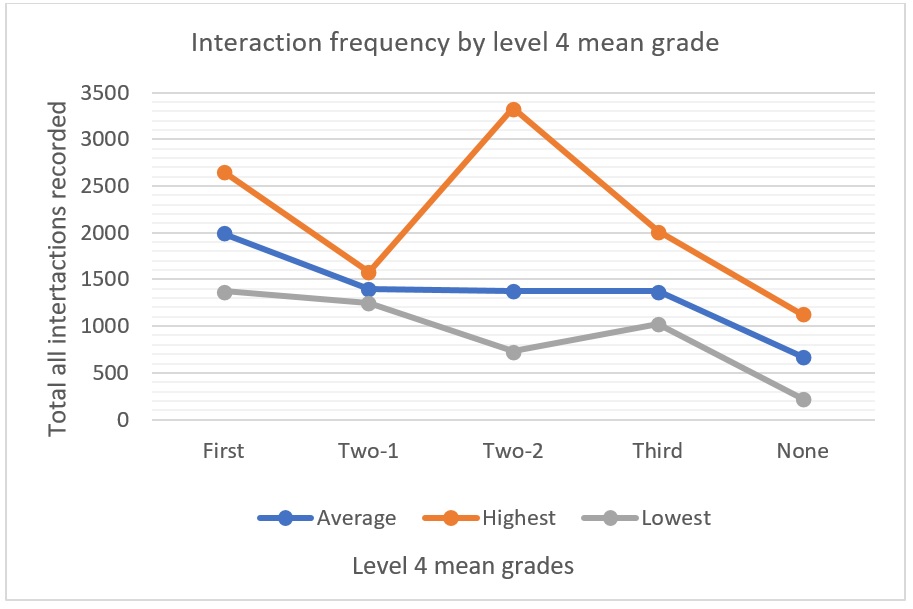
The average frequency of interactions is higher for those with the highest year end grades, reaching 1990 instances of recordable interaction for students whose year-end grade was a First. There was very little difference between the average frequency of interactions for students whose year-end grades were 2:1, 2:2 and Third (1398, 1375 and 1371 respectively). However, students ending the year at 2:2 had the largest range of interaction frequency. Students whose year-end grade was below classification had notably lower interaction frequencies, averaging 669 —two thirds fewer interactions than the average for students in the highest grade bracket. Whilst the averages do suggest a correlation between interaction frequency and academic outcomes, data at the individual level undermines predictive potential. The student with by far the most counts of interaction (3330) finished the year with a 2:2. One student who finished the year with a Third had more counts of interaction (2013) than the average for those gaining a First. One student whose year-end grade was below classification had more counts of interaction (1125) than did students who finished the year with a Third or 2:2.
These results would seem broadly to mirror those of (Summers et al., 2020) who compare activity and marks by quintile and found a correlation between attendance and VLE use frequency that is apparent from the first few weeks. Their interpretation of students with high frequencies of interaction but lower results is that they are working hard but unsuccessfully. We also cannot see the quality of these recorded interactions. It is entirely possible that a student might log on to the VLE and download all the materials provided. This student will therefore record fewer interactions than the student who logs on to the VLE each time they need information and searches for it anew. Likewise, when accessing E-resources, a student might download and save a selection of materials – and use them for the duration of the module – having searched effectively for material so needing to access fewer sources (or the same sources repeatedly). Another student might be less confident or efficient in their searching and storing of materials, so have to access E-resources more frequently. The latter may not be the most effective approach but would record a higher frequency of interaction. The implications, therefore, for the value of these LA data is that they do not capture what is meaningful; they are simply touch-point recordings that might actually mislead (Bassett-Dubsky, 2020). However, where they could add value is in making apparent less effective learning strategies where intervention can support improvement (Figure 2).
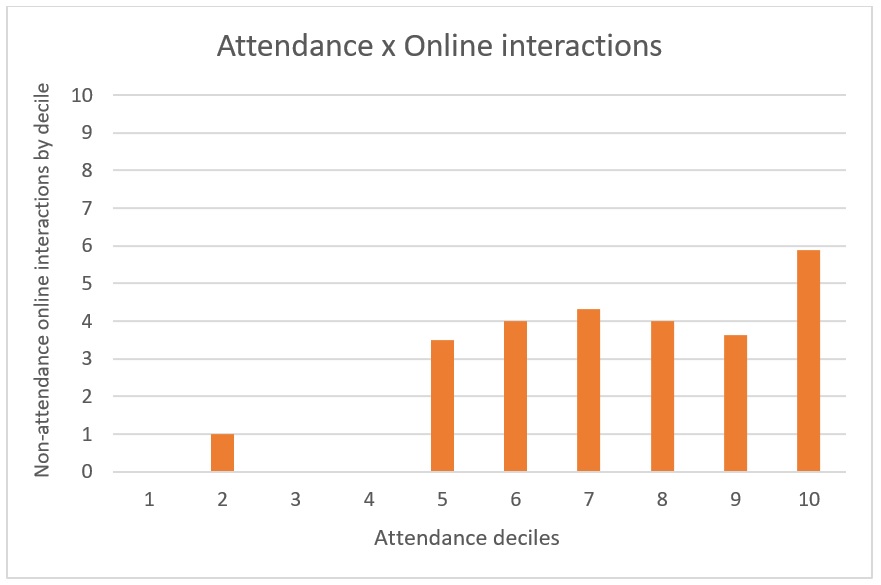
This suggestion seems further supported when the relationship between attendance and VLE logins/E-resource access is explored. At an overall average level, students in the highest attendance decile are also those who most frequently interact with online resources (Figure 2). However, at an individual level, Students in higher attendance deciles 9 and 10 appear to be in lower deciles for non-attendance interaction, whilst for numerous students in the lower attendance deciles the frequency of non-attendance interactions is greater than that of students with the highest attendance (Figure 3).
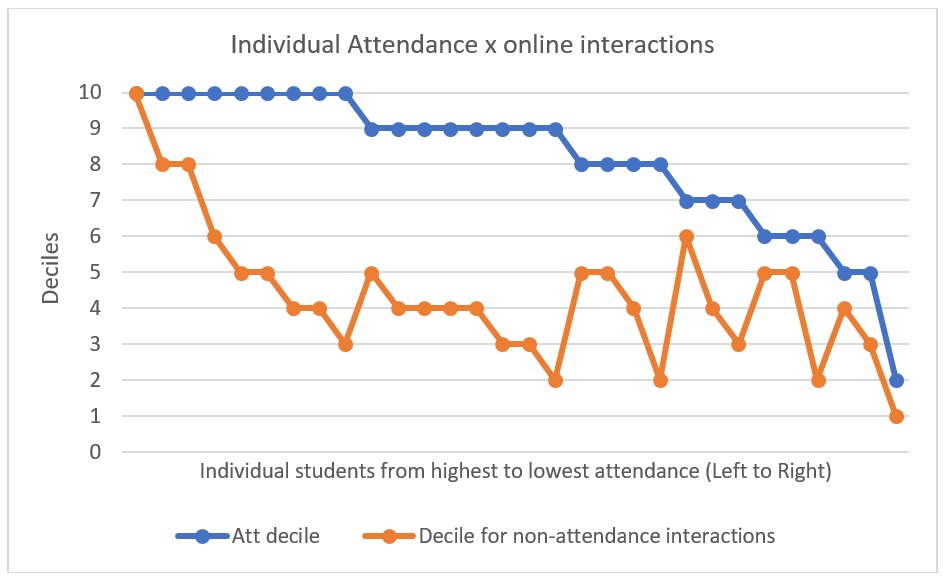
Lecture capture is not used at UON, and this pattern may reflect that seen in HEIs where lecture capture is used and access to lecture capture recordings may be used as an ineffective substitute for attendance (Dommett, Gardner, & Tilburg, 2019). It is possible that, in this study, the higher frequency of online interactions (VLE logins and E-resource use) might indicate more frequent online access in attempted compensation for non-attendance of taught sessions. Alternately, it might suggest that those who attend frequently are better able to navigate the online systems effectively so needing to log on less and downloading (or understanding sooner) the necessary materials.
Summers et al. (2020) found that the attendance of those in the lowest grade quintile declined from week 4 or 5, more significantly than that of students in higher grade quintiles. They also found that this attendance did not recover at the start of the second semester in the same way that it did for the majority of students. That steeper attendance decline for those working at the lowest grades was not so apparent in the data for this case study cohort, which may question the utility of this measure (in isolation) as a risk indicator.
First 4 wks attendance |
First 11 wks attendance |
Attendance over year |
|
---|---|---|---|
Students in lowest grade |
67% |
60% |
53% |
Students in highest grade |
100% |
98% |
96% |
This does suggest an important correlation between attendance and achievement. Looking at these first year data alongside the now available final year data shows that students who graduated with first class honours had, on average, the highest attendance at the start and end of their studies. However, there are multiple contradictions to this overall apparent trend. Whilst looking at year 1 attendance alone suggests a similar correlation between attendance and achievement for those who graduated with 2:1 and 2:2, this does not translate to attendance in the final semester of studies, where the average attendance for students who graduated with a 2:1 declined by 26% between year 1 and the final semester of study. Students who graduated with a 2:2 showed steady attendance between year 1 and the final semester of study (Table 3).
Exit classification |
Year 1 attendance |
S2 level 6 attendance |
---|---|---|
First |
94 |
88 |
Two-1 |
84 |
58 |
Two-2 |
75 |
75 |
There was no student whose attendance increased whose grades declined. However, decreases in attendance between level 4 and the final semester of study did not correlate with decline in grades. At an individual level, variation within those averages was such that any predictive potential would be seriously flawed (Figure 4).
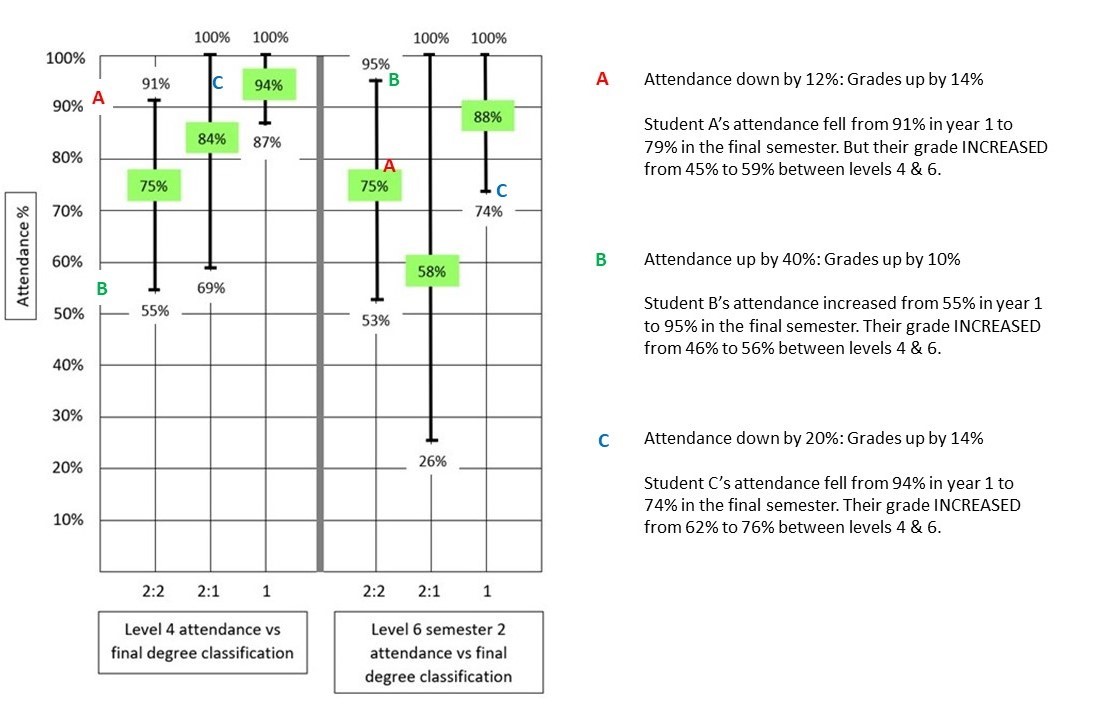
Nevertheless, of the students in the lowest grade bracket at level 4 (Table 2) , none of them have completed their studies. All bar one of those students withdrew or had their studies terminated prior to level 6. However, there were also students who withdrew or had their studies terminated (prior to or during level 6) who were averaging grades at Two-2 in level 4; so whereas it might not be true to say a student were ‘safe’ from withdrawal or termination if they were working at a certain level, it might be true to say that a student is particularly vulnerable to withdrawal or termination if they are working at a grade level that is unclassified during level 4. An additional (and sooner) indicator could be assessment extensions or fails in semester 1 level 4, as well as non-submission. None of the students who had extensions, fails or non-submissions in semester 1 level 4 graduated and all bar one withdrew or had their studies terminated prior to level 6. The strength of this indicator could only be seen with hindsight. That even an extension resulting in a pass, when it occurred in semester one, correlated with non-completion was something only recognisable once the standard programme completion date had passed.
Having established the risks of low academic attainment in semester one level 4, the correlation with academic success that was most noticeable was library resource use. Correlations between high academic achievement and library use have been noted previously (Robertshaw & Asher, 2019; Thorpe, Lukes, Bever, & He, 2016). However, the differences between E-resource access and physical library use are hard to account for and no system captures the use of physical library resources in the library when those resources are not loaned out. This means that physical resource use is particularly hard to recognise and appropriately value (Bassett-Dubsky, 2020).
AlthoughSummers et al. (2020) are somewhat dismissive of library use data, E-Resource access was not a data feed available to them through LA during their trial year. During this UON study, whilst no data feed was available for physical resource loans, E-resource access data were available (Figure 5).
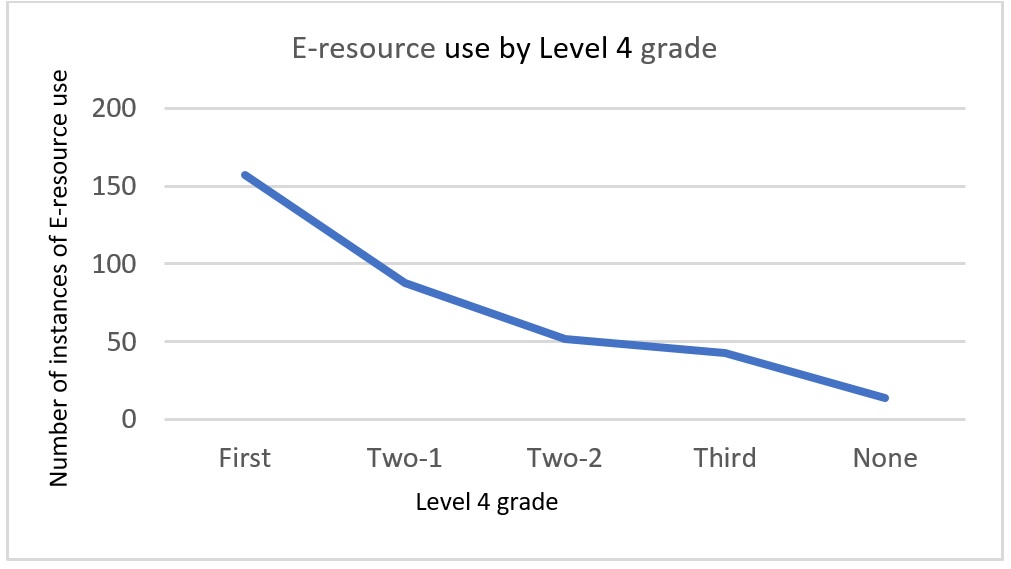
Looking at the 7 months between 1/10/2019 to 30/04/2020, there is a stark correlation between E-resource use and level 4 grade.
In isolation, how this information would be used to support students is unclear, since there is no indication of how the sources accessed are understood or used or if they are even read. If this information were set alongside the number of sources cited in students’ work and/or alongside tutor feedforward (so that suggestions to, for example, draw on wider literature, might be noted) then this information could have real potential, although workload implications could prove too great a barrier.
Relating L AD data to student engagement
As part of the UON case study, the participating cohort explored their understandings of what constituted engagement and what might be considered indicators of engagement. Four focus groups were scaffolded through use of a diamond 9 ranking activity, ranking ‘factors that indicate engagement’ from the most to least important. Both the concluding rankings of the 9 possible indicators and the discussions around the ranking were fruitful. As most relevant to this paper, the 9 options included attendance, VLE use, grades, meeting assignment deadlines and reading relevant and wider literature. Other options were 1-2-1or drop ins with learning support colleagues, time spend on campus outside scheduled hours, communicating with lecturers/personal tutor and determination to achieve and progress. The overall selection of options was determined based on the types of data available for LA and other factors the literature considered might suggest engagement.
The importance of literature use (indicated also by the E-resource correlations outlined above) was recognised by the students, being ranked 4th most significant (where 1 is most significant and 9 least significant). Students saw this as the most significant indicator of engagement from amongst the data types collated in the LAD.
It was seen as relevant to student engagement because it would feed into better grades. “If you’re determined to do well… you’re going to be reading as much as you can.” Wider research will support you “coming to lessons with more knowledge” so able to get more from those lessons. However, whilst the value of reading was agreed, there was indication that this benefit may not be fully accessible: "You can read as many books as you like but do you actually understand it?”
Attendance and VLE usage were jointly ranked of next highest significance to engagement. (At UON the VLE is referred to as NILE.)
Attendance was seen to relate to belonging "You get that social interaction… conversations that are broad"; as well as aiding learning through peer and tutor interaction: "It's not even just hearing what the lecturer has to say it's hearing the rest of the class discuss it, around it." "It might even change your own mind as well". However, it was not seen as necessary for engagement: "Attendance is not that important to be fair. And you're the one paying Nine Grand so I don't - stick it when I feel it." but more of an individual choice: "It just depends where you work best". Despite the belief in consumer/individual choice, there was also the idea that it might be harder to learn outside of class, just through VLE materials: "If you're here then the person's even more likely to engage in it but if you're just flicking through the slides you're like, yeah" "I get distracted".
However, when it came to using attendance as a proxy for engagement, all groups were aware of the need to appear engaged and also conscious that attendance could be quite an empty measure depending on what a person did once they were in attendance: "Some people aren't with it but they're attending… They're not really here". This recognition of performativity continued with the belief that visibility of VLE usage is “important cos then as a lecturer/personal tutor you can see that people are on [the VLE]." so generating those interaction touch points might compensate for being perceived as less engaged through attendance. VLE use was also seen as a proxy for attendance: “you can go on NILE when you're not attending” but then there were very contrasting views as to the value of the VLE, from low value: "If you know your thing then you don't really need NILE" to essential: "We need that. We're going on that like every day."
So it may be that the value of literature sources is known, but access (including understanding) is an issue. Whereas the value of the VLE is less clearly understood and under-appreciated and needs to be seen as valuable alongside lectures ie. they are both necessary and useful resources with their own, different merits. In programmes with professional standards, such as the one on which these students were enrolled, attendance is also vital for developing professional skills —not just in content but in developing professional practice (Oldfield, Rodwell, Curry, & Marks, 2018).
Earlier in this paper, some of the clearer indicators of risk to student outcomes (grades, progression and completion of studies) were level 4 grades averaging <39% and any assignment fails, extensions or non-submissions in level 4 semester one. The students in this study cohort did not consider grades to indicate engagement. A student might be fully engaged and still not achieve high grades. However, they did consider meeting assignment deadlines to indicate engagement. This was the students’ 2nd strongest indicator of engagement within the diamond 9 sort.
The most significant factor indicating engagement for the study cohort was a determination to achieve and progress. Having such a determination would indicate that the student were engaged. Much of the rationale for the higher rankings of meeting deadlines, communicating with lecturers and personal tutor and relevant and wider reading were because they evidenced that determination: "Like if you're determined to do well then you're going to be trying to get things done on time, you're going to be reading as much as you can". Staff would know students were engaged because they would see students were, "Using resources, reading." "Communicating" "Assignments on time. Being in your lectures, I guess". This is interesting, because it brings us back to much of the type of data that is captured within LA. "If you're not determined, your attendance is going to be bad, your grades are going to be bad like if you have no motivation… Without that [determination to progress and achieve] then nothing really gets done." However, as we have seen in the quantitative data analysed, whereas lack of determination and motivation is likely to lead to these indicators of poor attendance and grades; Poor attendance and grades do not necessarily indicate a lack of motivation.
Discussion
Students who are clearly trying to achieve (based on interaction frequency) but whose interactions are not seen to result in higher and increasing grades might be considered to be engaging, but not in ways that best support their progress. Constructive engagement might be defined as informed actions that support a determination to progress and achieve. However, a student might be determined to achieve and progress and taking actions that are less well informed so that their engagement and effort is high but not constructive (Bunce, King, Saran, & Talib, 2021; Freitas et al., 2015). To that extent, using LA does have the potential to recognise when measurable actions, that are likely to support constructive engagement, are not being taken (such as a student registering high attendance but low VLE and E-resource use). That is not the same as measuring engagement, but still offers potential for supporting engagement if LA are used with this understanding. It is the way we use LA that matters at an individual level (Herodotou, Rienties, Boroowa, & Zdráhal, 2019). Whilst LA may collate some useful indicators, predominantly descriptive data should not be used predictively or prescriptively. These are very different systems and far more complex (Susnjak et al., 2022). We need to embrace a variety of ways of working so that students have ownership of their own learning journey (Tobbell et al., 2021).
Of the descriptive data available to staff and students, the best early warning opportunity seems to be potential risk indicated by extensions, fails or non-submission in semester one level 4. Any semester one level 4 fail was indicative of risk regardless as to whether the student then passed on re-sit, yet it is the final grade (ie the re-sit grade) that is captured on LA. Extensions are not currently captured by LA and are not recorded in a format that can be directly transferred into ‘MyEngagement’. Similarly, if a student fails an assignment but passes the module overall, that fail will not be visible so that risk indicator will not be captured. If this granularity were captured, it would increase the potential of LA to support continuation and completion and reduce attrition.
These are concrete indicators that can inform constructive reaction through planned interventions before or at the start of semester 2. However, if this were handled poorly and these indicators were suggested as predictive of disengagement and drop out, then it would be easy to demotivate an already struggling student.Susnjak et al. (2022) suggest that it might discourage students to be mistakenly identified as being ‘at risk’ and thus lead to a self-fulfilling prophecy. However, that might also be true for students whose data suggest they really may be at risk. Examples of outliers in this context are therefore hugely important and we need to do more to understand what enables a student to ‘defy the odds’ in this way.
Average overall level 4 grades below a passing level should also trigger specific intervention beyond the opportunity for module re-sits. An average level 4 grade below the pass threshold indicates module re-sits in the next year of study. Ongoing and historical attendance data shows that attendance at taught sessions for students re-sitting modules is very poor and a further suggestion for future action would be to further explore how students do engage with re-sit modules and what actions they take to achieve a pass on re-sit and progress. Where practice includes split-level study, how can we better support students taking additional sessions and assignments from level 4 alongside level 5 modules, particularly where their overall credits studied are at the maximum allowable.
This paper is based on a small-scale study and makes no claims to generalisability. The scale of this study is seen as relevant to the way in which LA are used at the study HEI - at the level of the personal tutor who (in addition to the student) has access to the data. This is intended to help the student digest and process the information in the LAD with a supportive and context-aware tutor alongside them (Agudo-Peregrina, Iglesias-Pradas, Conde-Gonzalez, & Hernandez-Garcia, 2014); “to generate conversations, shared inquiry and solution-seeking” (Freitas et al., 2015). It would be useful to explore whether the most significant indicators of risk found at this programme level were also indicative in other programmes within the same division, and then across the same faculty. However, given the significant variation of programmes, wider generalisability cannot be assumed.
It should also be noted that systems implementation and approaches to using the data collated in those systems are two distinct areas of opportunity and/or concern. The focus of this study is on supporting the way in which available data (within and beyond LA) may be used. However, through ‘MyEngagement’ steering group participation, better awareness of which data might best be useful is feeding into ongoing improvement of LA as a system at this HEI. At a systems level, this is still subject to compatibility constraints and work is underway to capture additional risk indicators in compatible formats (Hanover-Research, 2016).
Conclusion
Although this is a small scale study, it does suggest the potential of LA to capture early insights that are relevant to students’ exit outcomes. Most significantly, combining the views of the study cohort about what they believed best indicated their engagement with an analysis of those same students’ early and exit data identifies two specific indicators of greatest relevance: E-resource use and meeting assignment deadlines.
Neither of those two specific indicators were or are captured (or reliably captured) through the current LAD in use at the study HEI. However, identifying their significance should support a re-prioritisation of the E-resource data feed – both in systems compatibility and in their contribution to the overall percentage of engagement presented in the LAD. It should also lead to a centralised capture of extension data (non-submission and fail data are already recorded in the VLE) and those data relating to missed assessment deadlines need to be captured together in a system that is compatible with the LAD. LA will have more credibility if these key indicators are available (and reliably so) because it will then reflect as ‘engagement’ what students themselves and the actual outcomes of students have recognised as indicative of engagement and its impact.
The interventions and responses suggested above are primarily academic, in a wider context where the complexities of students’ lives can make it challenging to take (or to take consistently) informed actions that support a determination to progress and achieve. The suggestions in the paper are based on use of LA and other available data and not exhaustive; a system of holistic support in all areas of student wellbeing is essential. There is a role for LAD in supporting that holistic system once the most relevant data are reliably available and combined. It can then meaningfully inform direct and personalised conversation with individual students, which should be located within a narrative of inclusion that focuses on removing barriers to engagement (Korhonen, 2012).